Is Knowledge Graph Just Another Means of Knowledge Visualization?
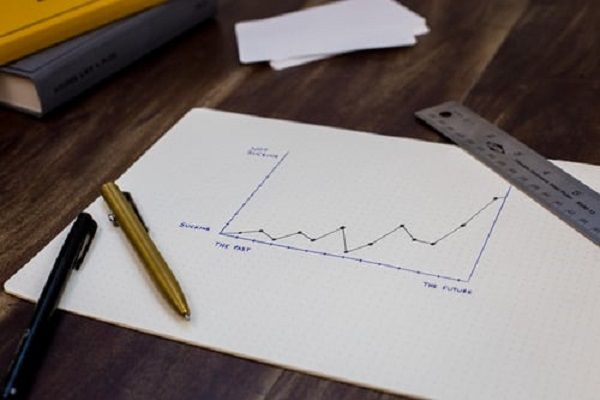
Organizations must deal with constantly changing data needs. They need to come up with newer and more efficient ways to assess and handle incoming data. Knowledge graphs help move from the traditional database to modern technologies such as machine learning, natural language processing, and semantics.
Knowledge graphs are the foundation for modern data and analytics. They help with data visualization. Combining machine learning and graph technologies has become a superior tool for businesses seeking to simplify data analysis.
However, the big question is whether knowledge graphs are just another means of knowledge visualization? We'll find out in this article. Additionally, we'll find out how knowledge graphs are solving machine learning problems and where they're applied in real-life situations.
What is a knowledge graph?
A knowledge graph is a network of real-world entities and how they relate to each other. Knowledge graphs link objects, events, and concepts to make sense of data in a given context. Information obtained from this network is critical in the visualization process for data analytics and inference.
A knowledge graph constitutes three primary components:
- nodes
- edges
- labels
A node refers to any object, event, or person, while an edge is a relationship existing between them.
For instance, if Facebook shares data with Instagram, the two parties are nodes, and their customer relationship is their edge.
A label is a name attached to the kind of relationship that the nodes in a network have at any given time.
How do knowledge graphs work?
Knowledge graphs constitute various data sets coming from different sources. These sources may differ in structure. Schemas, identities, and context create a system that puts together the diverse data to make sense.
Schemas provide a framework for knowledge graphs while the identities classify the involved nodes. The context gives an appropriate setting in which knowledge exists.
Using that structure to put this data together enables services like Google Search Engine to differentiate things with similar names but different identities. For instance, it cannot confuse Apple, the brand, with apple fruit.
Knowledge graphs use natural language processing power to create semantic networks. This is a comprehensive view of nodes, edges, and labels.
Upon reception of data, this network can identify individual objects and note their relationships. A complete knowledge graph can answer questions and enable search systems to retrieve and reuse old queries amidst growing information.
These features are part of the reason why the use of knowledge graphs in businesses for data analysis is increasingly becoming popular.
Knowledge graphs and visualizations
Knowledge visualization involves using visuals like graphs, sketches, images, and diagrams to present information.
Often there is confusion on whether knowledge graphs and knowledge visualization are the same things expressed differently. That view is true to some extent, but it is an oversimplification.
Knowledge graphs are not just another means of knowledge visualization but rather an elaboration of knowledge visualization.
Knowledge visualization on the other hand is different because it gives insight into data after its complete analysis. It presents the final phase of data processing.
However, knowledge graphs show the entire process from linking the nodes, establishing their relationship, sharing data, analysis, and visual presentation.
Essentially, knowledge visualization is a summarized portion of knowledge graphs.
Knowledge visualization needs knowledge graphs to be at the core of its strategy. Knowledge graphs can integrate heterogeneous data sources extensively due to their semantic networks. Knowledge visualization lacks such capability.
Knowledge graphs can use semantic enrichment knowledge to improve data quality and standardize it for a given entity. Such modified changes in data influence knowledge visualization because they change the appearance of data after its analysis.
Knowledge graphs as a solution to machine learning problems
The Cambridge Analytica scandal illustrates why modern machine learning methods need to become more transparent in their decisions.
To put it in context, that 2016 scandal tarnished the reputation of the American democratic process and over 200 others globally after the company confessed to influencing the voter's choices on the ballot.
Solutions like DARPA's explainable AI (XAI) program have been launched to create more trustable systems.
However, the explainable machine learning methods still fail to account for the users' context or background knowledge. This means it can be problematic to use these machine learning methods to control life-critical problems, such as healthcare.
Knowledge graphs help eliminate these shortcomings because they provide a valuable form of domain-specific, machine-readable knowledge through their semantic enrichment. Their connected datasets provide background knowledge for artificial intelligence systems to better explain their decisions to the users.
How knowledge graphs improve machine learning systems
Knowledge graphs improve machine learning systems' functionality in different ways.
First, they add context to data. The performance of machine learning improves with the incorporation of all the related data that an application needs.
By giving data, a context, knowledge graphs increase their informative value in a particular model. When machine learning understands the context of data, it can make better decisions. It now has a clearer picture of the factors associated with a given data's outlook and how they influence different functions.
Second, knowledge graphs combine several resources at once, as in search engines. Before knowledge graphs became part of Google's machine learning system, one had to search by typing all the text and symbols to find a given data source. But now, knowledge graphs have increased search engine prediction capabilities and made finding data online easier.
Where are knowledge graphs used today?
Knowledge graphs have a wide range of applications in the corporate and non-corporate worlds:
- They have improved browsing on search engines due to better predictability features.
- Knowledge graphs like KBpedia combine critical aspects of Wikidata and Wikipedia to help create an integrated online database for information sharing.
- They help track user connections on social media platforms like Facebook.
- In retail, knowledge graphs help recommend specific products to users based on their purchase behavior and market trends across specific demographic dynamics. For instance, Facebook knowledge graphs can recommend the purchase of noise-canceling headphones to an individual entering university grounds due to the popularity of the product there.
- They help entertainment platforms, such as Netflix, to recommend personalized content to users. Knowledge graphs track individuals' online information consumption and establish a relationship that pinpoints their favorite entertainment content.
- Knowledge graphs track fraud in the finance sector. They enable banks to understand money flow amongst their clients and note the non-compliant customers. Banks use this data to establish non-credit-worthy customers.
- In healthcare, knowledge graphs help organize health vocabularies and taxonomies for proper coding of medical bills. They also help organize medical research required to implement evidence-based practice in clinical settings. Besides, they enable healthcare providers to validate diagnoses and identify the treatment plans for each individual.
How are the knowledge graphs transforming organizations?
The most basic transformation impact of knowledge graphs to any industry relates to simplifying data analysis and visualization. When an organization embarks on a particular project, the leader must understand the why, what, where, who, and when associated with it. Information like this requires a thorough compilation of several forms of data, a capability that knowledge graphs can now facilitate.
Knowledge graphs reduce the number of assumptions that an organization has to work with when undertaking a particular project. The semantic enrichment network created by combining nodes and their edges ensures that data obtained achieves high precision.
Data processing occurs while considering the context, which helps in making better decisions. Thus, knowledge graphs are now the present and future of artificial intelligence systems.
That is so because AI needs to factor in data relationships and context to ensure precision. For instance, autonomous cars can utilize knowledge graphs to understand their environments and make better decisions to prevent traffic accidents.
Conclusion
A knowledge graph is not just another means of knowledge visualization. It is a more elaborate form of knowledge visualization. It combines data collection, processing, analysis, and presentation.
Knowledge graphs are becoming popular in machine learning because they combine several resources and add context to data. They make machine learning systems more precise in decision-making and trustable by considering the relationships between different data sources.
Knowledge graphs have become integral in business. Their ability to help create network linking nodes is significant to companies. It helps identify potential clients and target them for advertisements on social media platforms.
Knowledge graphs are transforming decision-making processes in businesses by widening their knowledge map and improving their overall functioning.
Happy learning!
Peer Review Contributions by: Onesmus Mbaabu
